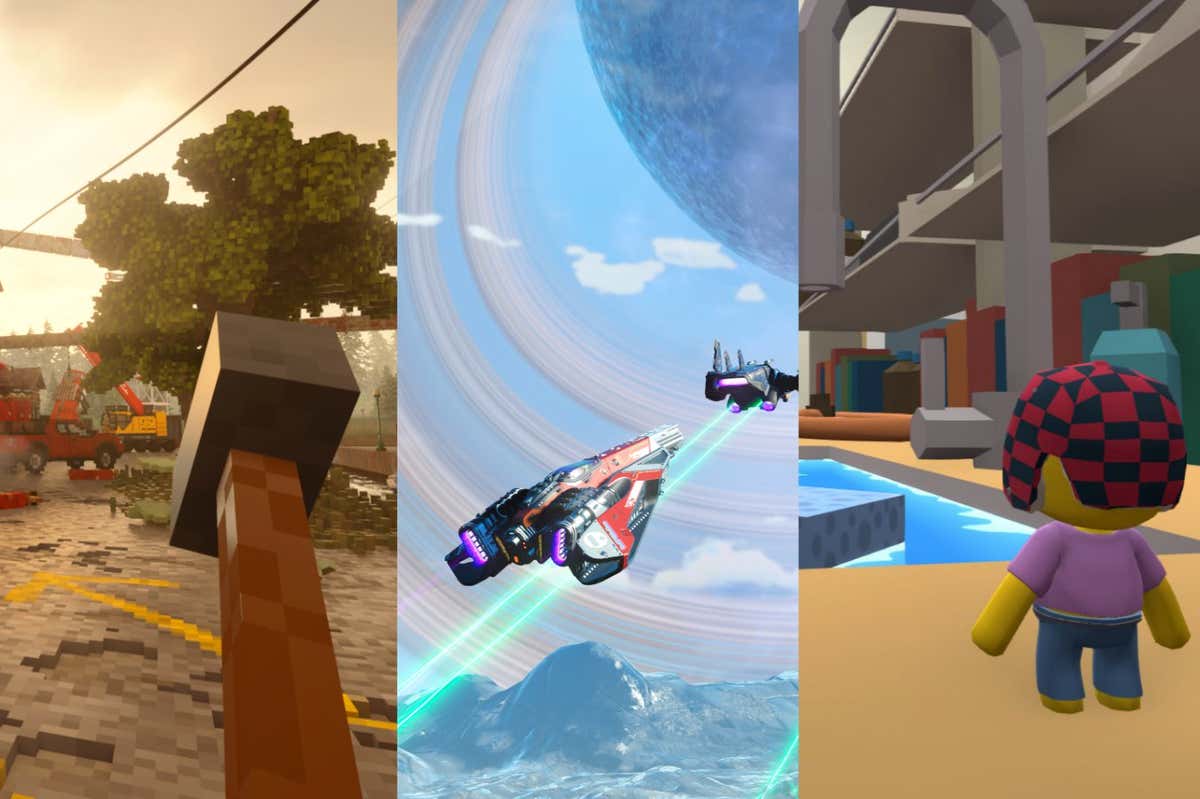
Some of the 3D virtual gaming environments that Google DeepMind’s SIMA AI has been mastering
SIMA Team
A Google DeepMind artificial intelligence model can play different open-world video games, such as No Man’s Sky, like a human just by watching video from a screen, which could be a step towards generally intelligent AIs that operate in the corporeal world.
Playing video games has long been a means of testing the progress of AI systems, such as Google DeepMind’s AI mastery of virtual chess and Go, but these games have obvious ways to win or lose, making it relatively straightforward to train an AI to succeed at them.
Advertisement
Open-world games with more abstract objectives and extraneous information that can be ignored, such as Minecraft, are harder for AI systems to crack. Because the array of choices available in these games makes them a little more like normal life, they are thought to be an important stepping stone towards training AI agents that could do jobs in the real world – such as controlling robots – and artificial general intelligence.
Now, researchers at Google DeepMind have developed an AI they call a Scalable Instructable Multiworld Agent, or SIMA, which can play nine different video games and virtual environments it hasn’t seen before using just the video feed from the game. This included the space-exploring No Man’s Sky, the problem-solving Teardown and the action-packed Goat Simulator 3.
“This is actually the interface that humans use to interact with a computer, it’s a very generic interface,” says Frederic Besse at DeepMind.
When asked in natural language, SIMA can perform about 600 tasks, of 10 seconds or less, that are common across the different games, such as moving around, using objects and navigating menus. It can also do more unique tasks like flying spaceships or mining for resources.
Besse and his colleagues used pre-existing video and image recognition models to interpret game video data, then trained SIMA to map what happens in the video to certain tasks. To provide this information, the researchers got pairs of people to play video games together, with one person watching the screen and telling the other what moves to make, and also made people watch back their gameplay and describe the mouse and keyboard moves they performed for their game actions. This allowed SIMA to learn how people’s descriptions of moves related to the tasks themselves.
When SIMA was trained on eight games, the researchers found it could then play a ninth game that it hadn’t seen before. However, it fell short of human-level performance. The researchers used a training method in which they rotated which eight games they trained the AI on so using the ninth game was the test, to ensure it can play any of the games it hasn’t seen before.
Extrapolating over different games is an important step towards a generalist AI agent, says Felipe Meneguzzi at the University of Aberdeen, UK, but SIMA can only currently perform a relatively limited set of short tasks that don’t require long-term planning. Performing a much wider range of complex tasks would be more difficult, he says.
“It’s worth remembering that for companies like DeepMind, this research isn’t really about games, it’s about robotics,” says Michael Cook at King’s College London. “Navigating 3D environments is a means to an end, and these companies are keen to make AI systems that can perceive and act in the world. So I don’t see this having a large impact on video games, but it might have a lot of unknown impacts on our life outside in the real world.”
Topics: