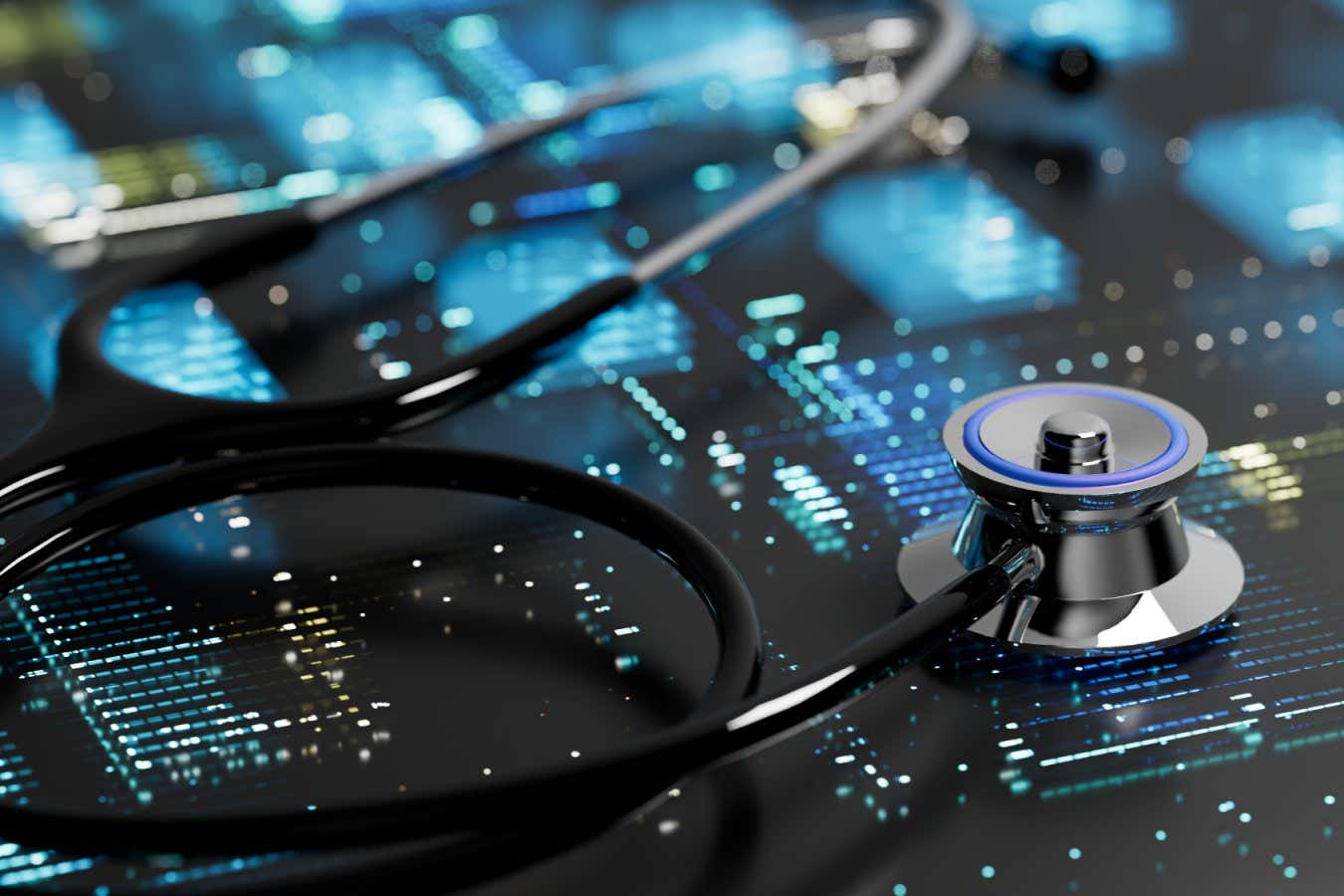
Don’t call your favourite AI “doctor” just yet
Just_Super/Getty Images
Advanced artificial intelligence models score well on professional medical exams but still flunk one of the most crucial physician tasks: talking with patients to gather relevant medical information and deliver an accurate diagnosis.
“While large language models show impressive results on multiple-choice tests, their accuracy drops significantly in dynamic conversations,” says Pranav Rajpurkar at Harvard University. “The models particularly struggle with open-ended diagnostic reasoning.”
Advertisement
That became evident when researchers developed a method for evaluating a clinical AI model’s reasoning capabilities based on simulated doctor-patient conversations. The “patients” were based on 2000 medical cases primarily drawn from professional US medical board exams.
“Simulating patient interactions enables the evaluation of medical history-taking skills, a critical component of clinical practice that cannot be assessed using case vignettes,” says Shreya Johri, also at Harvard University. The new evaluation benchmark, called CRAFT-MD, also “mirrors real-life scenarios, where patients may not know which details are crucial to share and may only disclose important information when prompted by specific questions”, she says.
The CRAFT-MD benchmark itself relies on AI. OpenAI’s GPT-4 model played the role of a “patient AI” in conversation with the “clinical AI” being tested. GPT-4 also helped grade the results by comparing the clinical AI’s diagnosis with the correct answer for each case. Human medical experts double-checked these evaluations. They also reviewed the conversations to check the patient AI’s accuracy and see if the clinical AI managed to gather the relevant medical information.
Multiple experiments showed that four leading large language models – OpenAI’s GPT-3.5 and GPT-4 models, Meta’s Llama-2-7b model and Mistral AI’s Mistral-v2-7b model – performed considerably worse on the conversation-based benchmark than they did when making diagnoses based on written summaries of the cases. OpenAI, Meta and Mistral AI did not respond to requests for comment.
For example, GPT-4’s diagnostic accuracy was an impressive 82 per cent when it was presented with structured case summaries and allowed to select the diagnosis from a multiple-choice list of answers, falling to just under 49 per cent when it did not have the multiple-choice options. When it had to make diagnoses from simulated patient conversations, however, its accuracy dropped to just 26 per cent.
And GPT-4 was the best-performing AI model tested in the study, with GPT-3.5 often coming in second, the Mistral AI model sometimes coming in second or third and Meta’s Llama model generally scoring lowest.
The AI models also failed to gather complete medical histories a significant proportion of the time, with leading model GPT-4 only doing so in 71 per cent of simulated patient conversations. Even when the AI models did gather a patient’s relevant medical history, they did not always produce the correct diagnoses.
Such simulated patient conversations represent a “far more useful” way to evaluate AI clinical reasoning capabilities than medical exams, says Eric Topol at the Scripps Research Translational Institute in California.
If an AI model eventually passes this benchmark, consistently making accurate diagnoses based on simulated patient conversations, this would not necessarily make it superior to human physicians, says Rajpurkar. He points out that medical practice in the real world is “messier” than in simulations. It involves managing multiple patients, coordinating with healthcare teams, performing physical exams and understanding “complex social and systemic factors” in local healthcare situations.
“Strong performance on our benchmark would suggest AI could be a powerful tool for supporting clinical work – but not necessarily a replacement for the holistic judgement of experienced physicians,” says Rajpurkar.
Topics: